QuantNet
Staff member
- Joined
- 10/23/03
- Messages
- 38
- Points
- 28
By EUAN SINCLAIR
The title of this piece was stolen. It was the title of a panel discussion at the 2010 MIT Sloan Sports Analytics Conference. It was no coincidence that the panel chair was Michael Lewis. He achieved initial fame as the writer of Liar's Poker, the story of the securitization of the mortgage market, then rose to greater prominence with the writing of Moneyball: The Art of Winning an Unfair Game, a case study of how the Oakland A’s overcame significant financial restrictions by applying statistical and economic principles to the analysis of baseball.
For a number of reasons (cheapening computer power, reduction in defense funded basic research and the availability of scientists from Russia, China and India) both finance and sports have increasing looked to quantitative techniques to find competitive advantage. In both fields the “geeks” are here to stay but there has also been a backlash against their presence. Some of this is easy to dismiss as ignorance and stupidity. It is also easy to understand the frustration of sports fans that saw sport as a way to escape math and thinking. A hobby is supposed to be fun and many people don’t find math fun. Fans are entitled to think that way. But management isn’t. It is their job to put a winning team on the field and if quantitative analysis can help them do that then they should embrace it or resign. Finance is also results oriented. It isn’t about having fun, so here the anti-quant backlash is a real problem.
But the geeks tend not to help themselves in this regard. As a finance geek myself, I have either done or have seen other geeks do things that hurt both their careers and the companies they work for. What follows is a partial list of the things that “geeks don’t get”.[prbreak][/prbreak]
1. Trading is a Business
This is the one principle that should guide all of your decisions. Making money is the goal. Solving interesting problems must be a means to this goal and not a goal in itself.
Never under-estimate the importance of seemingly mundane “business school” type jobs. Accounting, human resources, and legal services are all important. You don’t know exactly what they do but you couldn’t do these jobs and in many cases they will be more important to the success of a trading operation than the actual trading strategies. For example, Citadel has 15 billion dollars under management. It may have had a few years of poor returns, but most people would think this fund was highly successful as a business. Yet it does basically the same trades as hundreds of other trading firms and hedge funds. Indeed many of Citadel’s traders have been hired from these firms. So Citadel isn’t differentiated by its strategies at all. It is differentiated by its attention to all the “business school” stuff. Remember; a strategy is not a business.
2. Communicate
Research is only useful if it generates money. For this to happen, you need to be able to explain your results to traders and managers. There is no reason to get angry if you can’t explain your research to non-technical people. This is part of your job and if you can’t do it the fault is yours. You need to work on this just as you would work on any other technical shortcoming.
A particular way you can hurt your communication efforts is to get hung up on a technicality. Sooner or later, you will get very exited to “discover” a flaw in a commonly used piece of mathematics. For example, this could be the Sharpe ratio, correlation, Value at Risk or the implied volatility curve. You will be excited for good reason: you probably have found a discrepancy between the real meaning of these ideas and the common understanding of them. You may also think you are the first to have noticed the particular shortcoming. But don’t make this discovery a focus of a presentation. I’m not saying that most traders really understand Sharpe ratios, correlation or VAR. They generally don’t. But they probably understand enough for their needs. People need to be told broad ideas, not details. The fact that a Sharpe ratio doesn’t distinguish between downside and upside deviation is unlikely to be the crucial thing in initially deciding whether to go further with a strategy. If a manager signs off on the main idea, he can then ask a more technical person to go through things more carefully. Speak to the level of your audience.
Remember that you will never know what someone else knows. Other people may have a different way of understanding the world. This is a good thing. You should hope that your colleagues have a complementary set of skills to your own. No matter the temptation, don’t assume that a non-quant is stupid. Someone once said something to the effect that if you find a field of human endeavor where everyone is an idiot, you have probably misunderstood the situation.
3. Simplicity is a Virtue
Always use the simplest method possible. This ties in closely with my first point: trading is a business. Problems are to be solved, not treated as sources of amusement or intellectual challenges. Brute force is often a perfectly acceptable technique.
The worst examples of over-complicating problems occur when a quant applies a favorite technique that is simply not suited to the particular situation. Differential geometry and Lie groups are not needed to solve the Black Scholes Merton equation.
4. The Model is not Reality
Money is made by trading instruments, not by analyzing models. Models are a way to simplify the world but they are not actually the world themselves. It should (but doesn’t) go without saying that you need to understand the assumptions behind your models, but you must also remember what impudence is involved in even trying to create financial models in the first place. Any such modeling will necessarily involve enormous simplifications.
I was recently observing an online discussion with the author of a book about high frequency trading. The writer defended a point by stating, “ have you never heard of Brownian motion as a model for diffusion of asset prices? Longer horizons are always less certain than the shorter ones.” This is not a statement about markets. This is a statement about a particular model (a number of situations are more certain in the long run). To be completely fair, the author could have been referring to a feature of the model, but the statement easily leads the reader to infer otherwise. Such blurring of the distinction between models and reality is far too common.
Conclusion
As a geek you will have a number of useful quantitative skills and will probably be an intelligent person. But these skills have to be used as tools to make money rather than cherished for their own sake. Ideally, I would like to see “quant” used as a name for an approach to markets rather than as a job title. What is important about quantitative trading isn’t the math, it is the trading.
About the Author:
Euan Sinclair is an option trader with over 15 years of experience trading professionally. He specializes in the design and implementation of quantitative trading strategies. Sinclair is currently a proprietary option trader for Bluefin Trading, where he trades based on quantitative models of his own design. He holds a PhD in theoretical physics from the University of Bristol. Euan Sinclair is author of Volatility Trading and an upcoming book Option Trading Volatility Strategies Techniques.
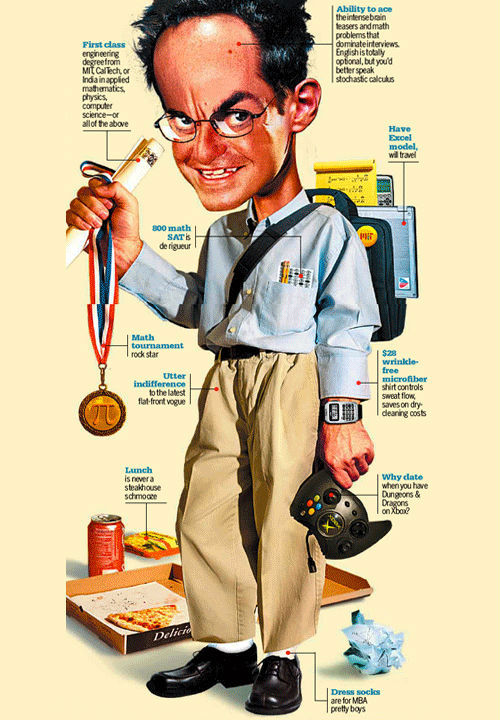
For a number of reasons (cheapening computer power, reduction in defense funded basic research and the availability of scientists from Russia, China and India) both finance and sports have increasing looked to quantitative techniques to find competitive advantage. In both fields the “geeks” are here to stay but there has also been a backlash against their presence. Some of this is easy to dismiss as ignorance and stupidity. It is also easy to understand the frustration of sports fans that saw sport as a way to escape math and thinking. A hobby is supposed to be fun and many people don’t find math fun. Fans are entitled to think that way. But management isn’t. It is their job to put a winning team on the field and if quantitative analysis can help them do that then they should embrace it or resign. Finance is also results oriented. It isn’t about having fun, so here the anti-quant backlash is a real problem.
But the geeks tend not to help themselves in this regard. As a finance geek myself, I have either done or have seen other geeks do things that hurt both their careers and the companies they work for. What follows is a partial list of the things that “geeks don’t get”.[prbreak][/prbreak]
1. Trading is a Business
This is the one principle that should guide all of your decisions. Making money is the goal. Solving interesting problems must be a means to this goal and not a goal in itself.
Never under-estimate the importance of seemingly mundane “business school” type jobs. Accounting, human resources, and legal services are all important. You don’t know exactly what they do but you couldn’t do these jobs and in many cases they will be more important to the success of a trading operation than the actual trading strategies. For example, Citadel has 15 billion dollars under management. It may have had a few years of poor returns, but most people would think this fund was highly successful as a business. Yet it does basically the same trades as hundreds of other trading firms and hedge funds. Indeed many of Citadel’s traders have been hired from these firms. So Citadel isn’t differentiated by its strategies at all. It is differentiated by its attention to all the “business school” stuff. Remember; a strategy is not a business.
2. Communicate
Research is only useful if it generates money. For this to happen, you need to be able to explain your results to traders and managers. There is no reason to get angry if you can’t explain your research to non-technical people. This is part of your job and if you can’t do it the fault is yours. You need to work on this just as you would work on any other technical shortcoming.
A particular way you can hurt your communication efforts is to get hung up on a technicality. Sooner or later, you will get very exited to “discover” a flaw in a commonly used piece of mathematics. For example, this could be the Sharpe ratio, correlation, Value at Risk or the implied volatility curve. You will be excited for good reason: you probably have found a discrepancy between the real meaning of these ideas and the common understanding of them. You may also think you are the first to have noticed the particular shortcoming. But don’t make this discovery a focus of a presentation. I’m not saying that most traders really understand Sharpe ratios, correlation or VAR. They generally don’t. But they probably understand enough for their needs. People need to be told broad ideas, not details. The fact that a Sharpe ratio doesn’t distinguish between downside and upside deviation is unlikely to be the crucial thing in initially deciding whether to go further with a strategy. If a manager signs off on the main idea, he can then ask a more technical person to go through things more carefully. Speak to the level of your audience.
Remember that you will never know what someone else knows. Other people may have a different way of understanding the world. This is a good thing. You should hope that your colleagues have a complementary set of skills to your own. No matter the temptation, don’t assume that a non-quant is stupid. Someone once said something to the effect that if you find a field of human endeavor where everyone is an idiot, you have probably misunderstood the situation.
3. Simplicity is a Virtue
Always use the simplest method possible. This ties in closely with my first point: trading is a business. Problems are to be solved, not treated as sources of amusement or intellectual challenges. Brute force is often a perfectly acceptable technique.
The worst examples of over-complicating problems occur when a quant applies a favorite technique that is simply not suited to the particular situation. Differential geometry and Lie groups are not needed to solve the Black Scholes Merton equation.
4. The Model is not Reality
Money is made by trading instruments, not by analyzing models. Models are a way to simplify the world but they are not actually the world themselves. It should (but doesn’t) go without saying that you need to understand the assumptions behind your models, but you must also remember what impudence is involved in even trying to create financial models in the first place. Any such modeling will necessarily involve enormous simplifications.
I was recently observing an online discussion with the author of a book about high frequency trading. The writer defended a point by stating, “ have you never heard of Brownian motion as a model for diffusion of asset prices? Longer horizons are always less certain than the shorter ones.” This is not a statement about markets. This is a statement about a particular model (a number of situations are more certain in the long run). To be completely fair, the author could have been referring to a feature of the model, but the statement easily leads the reader to infer otherwise. Such blurring of the distinction between models and reality is far too common.
Conclusion
As a geek you will have a number of useful quantitative skills and will probably be an intelligent person. But these skills have to be used as tools to make money rather than cherished for their own sake. Ideally, I would like to see “quant” used as a name for an approach to markets rather than as a job title. What is important about quantitative trading isn’t the math, it is the trading.
About the Author:
Euan Sinclair is an option trader with over 15 years of experience trading professionally. He specializes in the design and implementation of quantitative trading strategies. Sinclair is currently a proprietary option trader for Bluefin Trading, where he trades based on quantitative models of his own design. He holds a PhD in theoretical physics from the University of Bristol. Euan Sinclair is author of Volatility Trading and an upcoming book Option Trading Volatility Strategies Techniques.