- Joined
- 4/24/07
- Messages
- 11
- Points
- 13
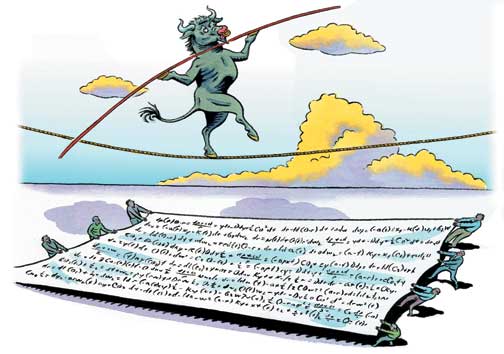
We are the CDO makers,
and we are the dreamers of dreams.
Betting on lone loss triggers,
and trading in eclectic teams.
Spread losers and yield forsakers,
for whom the pale bonus gleams.
Yet we are the sole underwriters,
of the deals, forever it seems!
Fantasia on a Theme by William Blake
[prbreak][/prbreak]All over the world, it has become fashionable for Universities and Colleges to offer Masters degree programs in quantitative finance or financial engineering (FE), a code word meaning the solution of the Black-Scholes option pricing differential equation in as many ways as possible. To do so, students are taught to use basic techniques in numerical analysis whenever the equation is either non-linear or does not lend itself to the standard analytical solution. As a precursor to this main task, the program usually includes a course in stochastic calculus during which Ito's celebrated lemma is discussed, proved and used.
In general, the cost and length of such programs are remarkably similar despite the variability in the quality of the teachers and the brand name of the institution. In many cases the FE program is one of the biggest money makers at the University, if not the biggest, enabling schools to charge somewhere between $25,000 and $35,000 for eighteen months of night classes taught by top professionals in the field. Many such people are in fact refugees from bulge-bracket Wall Street firms looking for something to do before heading out to pasture in Florida and Arizona. In addition to being crassly commercial in their approach to knowledge transfer, often resorting to advertising their own former firm in the classroom, they are willing to accept much less in compensation than full-time professors and never become management headaches to the institution. Even Ivy League schools like Princeton University, who swore up and down they would never play this game, are now happily teaching finance and deriving significant incremental income from a fully depreciated curriculum.
The techniques taught in quantitative finance are completely standard in other fields. In most cases, the only exciting thing about the curriculum is that one day these methods might be applied on Wall Street to the calculation of cash flows. If they were instead applied to the making of widgets or the collection of tomatoes, it is a fair bet that nobody would be interested in them, and certainly no university would be able to charge $35K to learn them. In many other cases, schools with no MBA program have succeeded in manifesting an MBA curriculum out of thin air under the FE banner. At this point, financial engineering does not appear to have any specific meaning, or perhaps it means whatever it takes to get people through the door.
It is a plain fact that the field of quantitative finance has not made a single fundamental step forward over the past twenty years, not to mention that Black himself, by his own admission, had nothing to do with the equation that now bears his illustrious name. The BS equation was first formulated and solved by Casey Sprenkle some ten years before Black's famous 1973 paper in the Journal of Political Economy. Regrettably, it is still politically incorrect to give due credit to someone who made a real contribution to finance. Unlike those of some of his associates, Black's reputation hardly hangs on one paper.
Statistics and numerical analysis have nothing to do with finance per se but are merely tools of financial analysis, just like accounting statements and legal opinions. Finance is quantitative by definition; there is thus no need to add an adolescent adjective to the word. This is like saying aerial flight or wet swimming. Although people employed as aerospace engineers use computers on a daily basis, none would describe him- or herself as a computer programmer.
But if this were about mere semantics, it would not be worth mentioning. Unfortunately, FE programs are also drifting farther and farther away from their purported subject matter. In effect, quantitative finance has entered the scholastic stage whereby numerical techniques are taught completely out of context as if a deal were somehow a differential equation that could be solved for the right solution. In fact, there is no solution to a deal as there is to a differential equation. At this point, analysts are talking about investing angels dancing on financial pins. Even worse, professional societies devoted to financial engineering are in reality pressure groups acting on behalf of various financial constituencies, like hedge fund managers seeking to get the regulators off their proverbial backs. Although every American citizen has the right to lobby whomever he pleases, this cannot exactly be described as furthering the field of financial engineering or building esprit de corps among its members.
Students thinking themselves financial experts simply because they can solve the BS equation in a few minutes (there is apparently no other one around) are being misled by their own mentors and teachers into the naïve belief that this amounts to finance. Seminars with magical titles like How I Became a Quant or A Quant Roundtable only serve to perpetuate a myth, the myth that finance is about differential equations or positive definite matrices. Anyone even remotely acquainted with the practice of finance knows full well how far removed such mathematical topics truly are from the real subject matter and the day-to-day bubbling within the cauldron of finance.
A deal only happens when various constituencies (lawyers, investors, bankers, rating agencies sometimes, regulators, accountants, etc.) are able to come together under a unified framework. This is not the time to deliberate or lecture on cross-correlation and conditional VAR. On the contrary, any such talk is completely counter-productive and propagates the negative stereotype commonly attached to those that engage in it to impress or intimidate their neighbors. Rather than repel them, a savvy financial engineer ought to find a way to bring all the parties together, thereby placing him- or herself at the center of the deal instead of its margins. However, this pre-supposes the emergence of a new language better suited to deal making, i.e. not the current one extracted lock, stock and barrel from theoretical physics. To be on a fantastic journey is great only as long as everybody else is on board with you.
Too often, students who would otherwise have something valuable to contribute are being led down the primrose path at the instigation of people for whom this is, at best, a hobby. The promise of high-flying jobs in New York is all that has propped enrollment at its current levels, since once on the job, graduates of financial engineering programs quickly become aware that it is MBA graduates, and not themselves, who are destined to breathe the rarefied air of boardroom deal-making. In fact, the label quant has now become quasi-pejorative, the practical equivalent of geek or inconsequential number-cruncher. Deal-makers do not want such people in front of their clients, if only for fear of hearing naïve prognostications of hetero-scedasticity and Gaussian copula bandied about before befuddled investors (and even before lunch).
Not surprisingly, business school professors often warn their students about not being labeled a quant if they ever want a career in the community of finance. Given the current state of affairs, we could not agree more. Every financial professional worth his salt should be numerate at least to the extent necessary to do his or her own deals, and ideally more. If solving a differential equation is what it takes, then so be it. Unfortunately, it rarely if ever does. Professor Joel Hasbrouck of the Stern School of Business at New York University has posted a rather blunt Power Point presentation entitled Quantitative Finance on the Internet. Despite the obviously self-serving and competitive nature of his remarks, he is largely and sadly correct.
Many students pursuing financial engineering degrees already have technical degrees in other fields and are merely looking to acquire a brand name enabling them to join the party on Wall Street and earn much more than they would in their own field, assuming they would even find a job there. For them, most of the course work in the program is useless or repetitive at best, since they already know what they need to know to perform. Others are liberal arts majors with essentially no background in numerical analysis. They find the program hard and commonly team up with students from the first category to make sure they can do all the assignments. To such students, names like Crank-Nicholson and McCormack easily acquire mythical status, being by definition the way to solve the BS equation numerically. They have no way to gauge whether these are just two methods among many or the pure, unadulterated truth.
In some cases, students are blindly taught to use techniques that only work because the BS equation is parabolic. The course assignments we have looked at are remarkably commercial in intent and mainly serve to stifle basic creativity by intimating, for instance, that what Risk Metrics (a commercial vendor of analytical services) does is the right way to model credit or market risk. This is thoroughly and sleazily transparent, anti-intellectual and only fosters cynicism in the student body when it is perfectly clear that there is no such thing as a right answer in finance, unless by that term one trivially means that no logical mistakes have been made.
What is obvious and regrettable is that no effort is ever made to teach numerical analysis as a proper and rigorous discipline. Instead, students literally learn numerical recipes and are no more equipped to handle reality than someone equipped with a driver's license when their car breaks down. One also gets the disturbing feeling that the majority of teachers involved in quantitative finance have limited knowledge of either finance or of the elements of numerical analysis. Unfortunately, too many professors in that field are there for the same reason that the school can charge enormous tuition fees, i.e. they can earn much more for teaching the same material in finance than they would in the original field. It's hard to turn down $150,000 for teaching either statistics or the numerical solution of partial differential equations on a full-time basis when your equally competent buddies from graduate school are doing the same thing elsewhere for $50,000.
Although we freely admit that we have not performed the monumental task of a complete inventory and comparative analysis of financial engineering curricula, a cursory review of the most popular ones reveals what seems to be their central dilemma, which is how to fill 18 months of teaching with a single topic: stochastic calculus and its applications. The general answer appears to be to fill the remaining 14 months or so with subsidiary material peripheral to finance but available for much less elsewhere. Here, the main target is numerical analysis. In the latter domain, although students are taught a few useful techniques, the elements of numerical analysis are not addressed, perhaps because this would take up too much time and force the school to hire teachers who would demand higher pay and thus decrease yields.
The upshot is that the curriculum is always sitting between chairs but never on any one of them. One meanders across finance discussing swaps, default swaps and various options (instruments that hardly require Herculean intellectual prowess to grasp), engages in endless and meaningless debates on the eigenvalues of correlation matrices, and then dabbles in numerical analysis by learning basic methods applied to the BS equation taken as the last word in finance for the remainder of mankind's existence. At no time, as far as we can tell, are students taught how to construct a numerical method from scratch or how to tell if it will work or fail.
Throughout our Internet search, the following topics were absent from the syllabi of the numerical analysis courses within the financial engineering curricula of the academic institutions we reviewed:
- Z-transforms and Laplace transforms
- Banach and Sobolev spaces
- Fourier series and transforms (one exception)
- Lax equivalence theorem (same exception)
- Von Neumann stability analysis
- Courant-Friedrichs-Loewy (CFL) condition
- The Nyquist sampling theorem (useful in Fourier analysis)
- Convergence analysis
- Error propagation analysis
- The Weierstrass approximation theorem
- The interplay between truncation and discretization error
The consequence of all this is that today, and through no fault of their own, students with degrees in financial engineering are ill-equipped to face the rapidly changing face of finance. Once ensconced in their jobs, they are quickly marginalized and relegated to the role of glorified programmer until being eliminated in the next headcount reduction because (with unfortunate justification) they are not considered producers.
It would be a different matter if financial engineering were just a code word for numerical analysis with finance used as a marketing mechanism to attract people to the field. It would be equally acceptable if financial engineering were devoted to the actual practice of finance instead of being largely an obsession with one equation, no matter how interesting it might be. Someone who has never done an actual deal can hardly be expected to know how deals are done, let alone teach how to do them. Likewise, a manager who used to supervise twenty-five Ph.D.s in some research department on Wall Street has as much knowledge about deal making as an usher at Yankee Stadium has about baseball. On the contrary, it has become painfully obvious that these managers, if the term can be used at all to describe this level of incompetence, are precisely the people who truly need supervision instead of underlings who, at bottom, never make a single decision that could take their firm down.
Financial engineering never grew up within finance; it was taken over by physics. This is not surprising given that the same thing happened to economics 100 years ago. Unless the field re-invents itself pronto and starts becoming relevant to what people actually do out there, graduates with newly minted financial engineering degrees hoping to see a decent return on their own or their parents' sizable investment will continue to be sorely disappointed by their actual career prospects, and will keep wondering where in God's name they went wrong.Regrettably, the answer is: nowhere.
Sylvain Raynes is a founding principal of R&R Consulting, a structured credit metrics consultancy founded in 2000. He co-authored The Analysis of Structured Securities: Precise Risk Measurement and Capital Allocation with Ann Rutledge, the other R in R&R Consulting. Their second book Elements of Structured Finance is scheduled for release in June 2010.
As an Amazon Associate we earn from qualifying purchases.